About
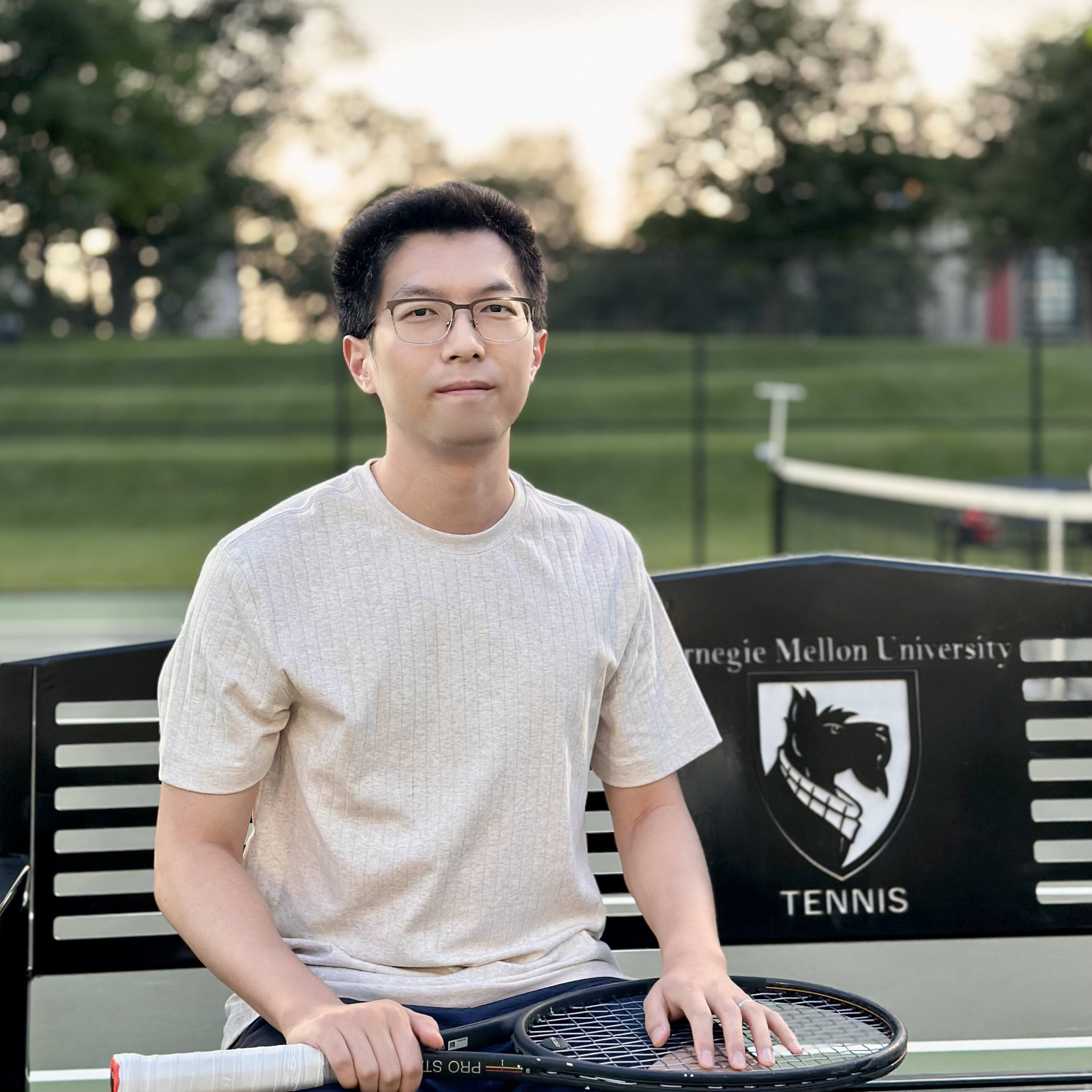
Peide Huang
I am a final-year Ph.D. student advised by Prof. Ding Zhao@SafeAI Lab at Carnegie Mellon University. I am also fortunate to work with Prof. Fei Fang@AI and Social Good Lab at CMU. Prior to joining CMU, I received my Bachelor's degree from Nanyang Technological University, Singapore and Master's degree from Stanford University.
My research goal is to understand the interplay between the learning agent and the tasks, with the objective to enable safe, robust, and efficient decision making. To achieve this goal, I leverage curriculum learning, game theory, and foundation models. I also tackle real-world applications in robotics and autonomous driving.
I am open to collaboration. If you are interested, please contact me via email.
Email  /  CV  /  Google Scholar  /  Twitter
News
- 11/2023: Our RoboTool is covered by TechXplore as a featured story!
- 08/2023: Two papers (one Oral) are accepted by CoRL 2023!
- 04/2023: I was awarded a fellowship from the CMU Machine Learning Department.
- 01/2023: Group Distributionally Robust RL is accepted by AISTATS 2023!
- 10/2022: I received Top Reviewer Award and Scholar Award from NeurIPS 2022 Committee.
- 09/2022: GRADIENT is accepted by NeurIPS 2022! Thanks to all the co-authors!
- 06/2022: Our work on accelerated policy evaluation in the presence of rare events is accepted by IROS 2022.
- 04/2022: My first conference paper is accepted by IJCAI 2022. Thank all the co-authors!
Publications
* indicates equal contribution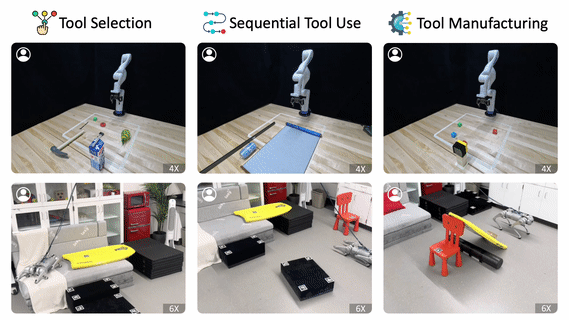
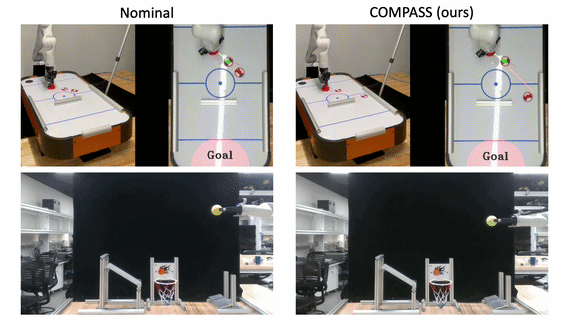
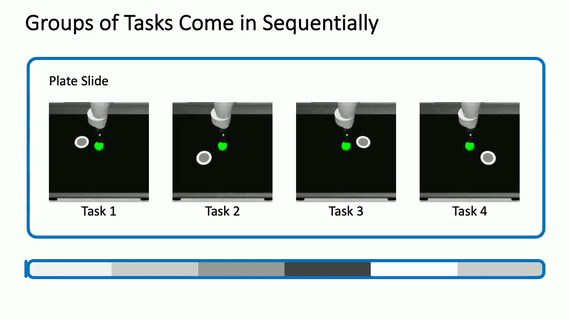
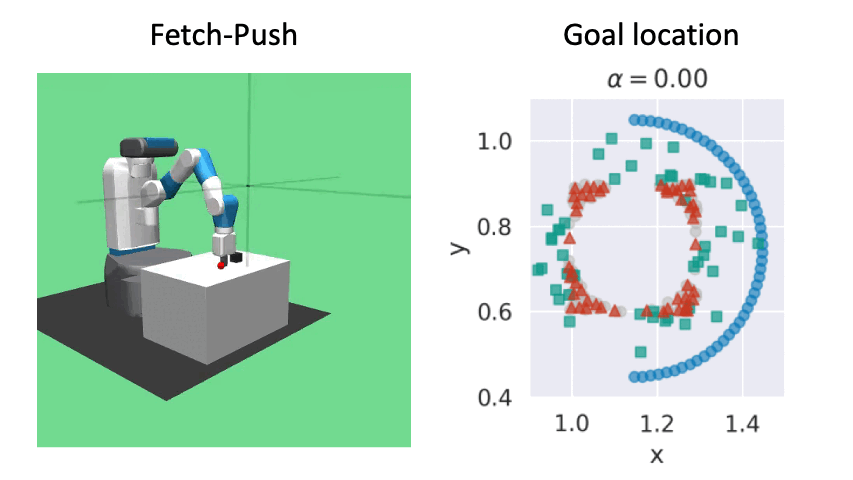
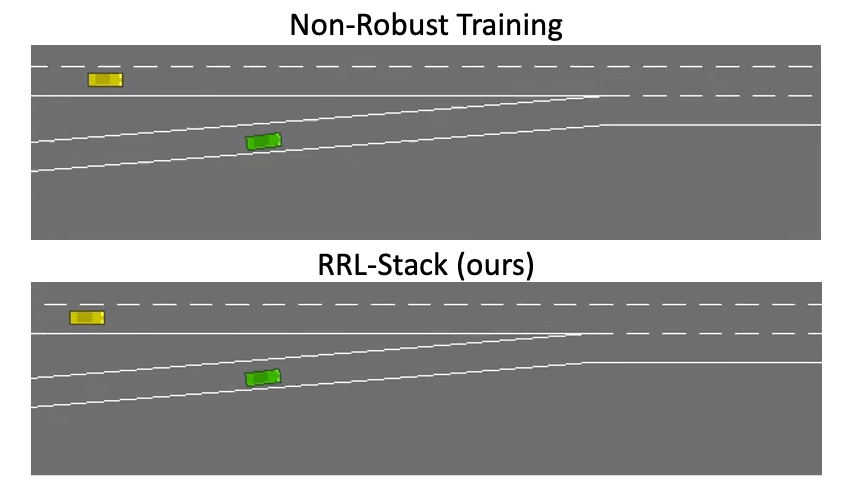
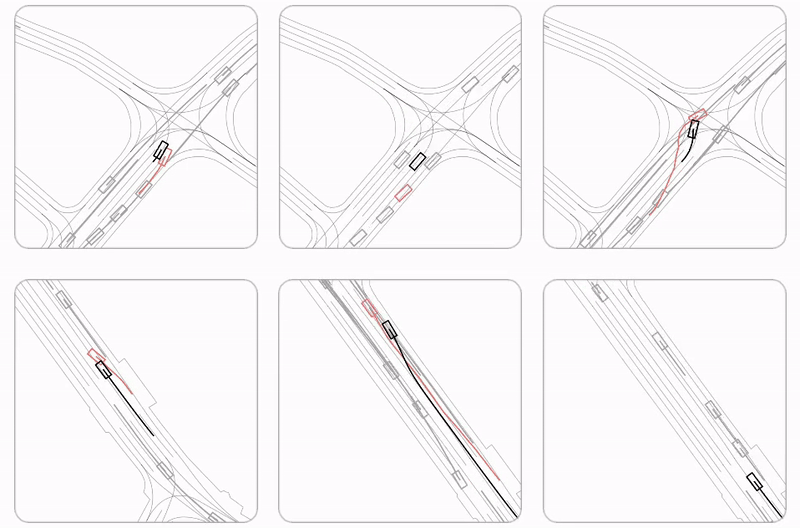
CaDRE: Controllable and Diverse Generation of Safety-Critical Driving Scenarios using Real-World Trajectories
Peide Huang,
Preprint
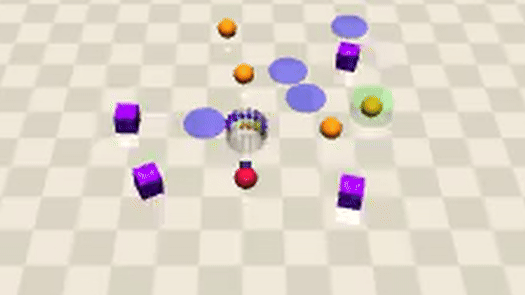
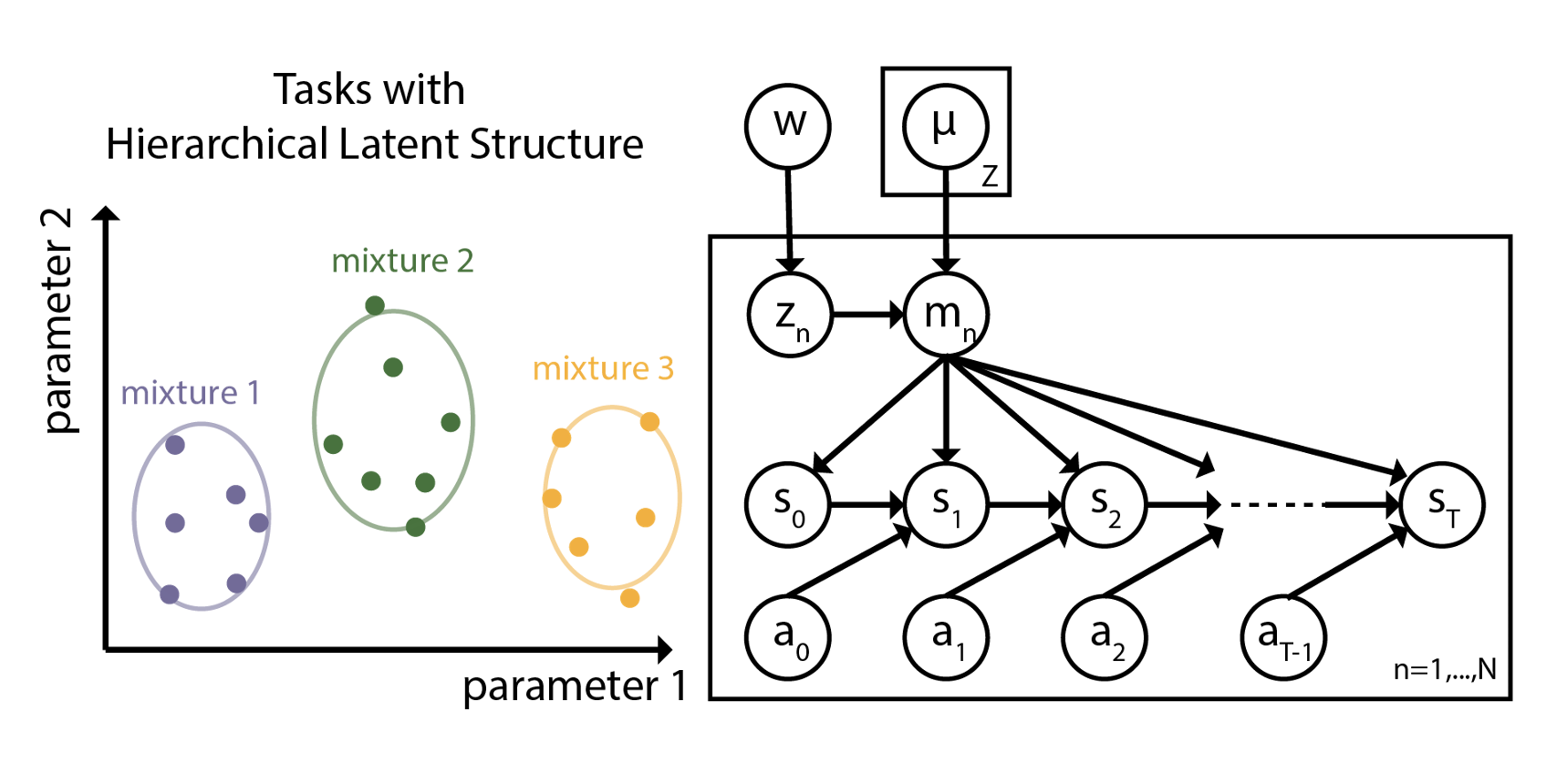
Group Distributionally Robust Reinforcement Learning with Hierarchical Latent Variables
The 26th International Conference on Artificial Intelligence and Statistics (AISTATS 2023)
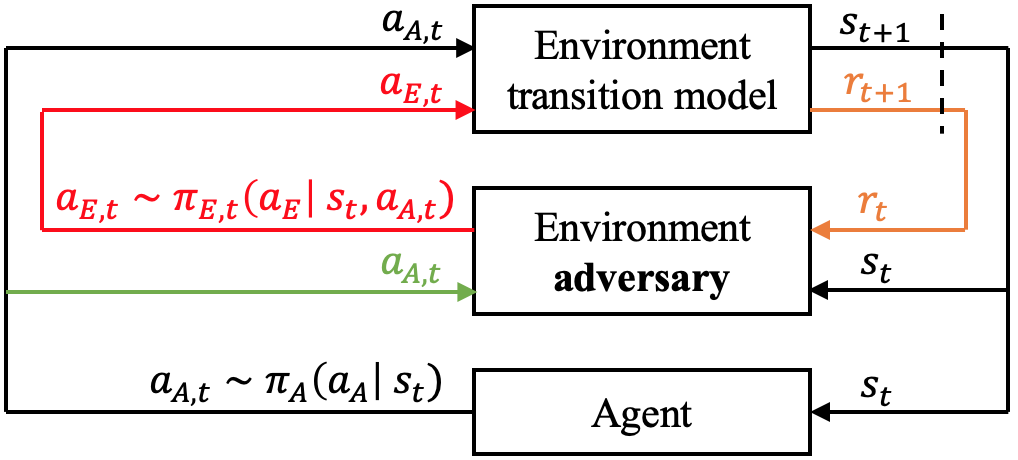
Scalable Safety-Critical Policy Evaluation with Accelerated Rare Event Sampling
2022 IEEE/RSJ International Conference on Intelligent Robots and Systems (IROS 2022)
Abridged in ICLR 2021 Workshop in Security and Safety in Machine Learning Systems
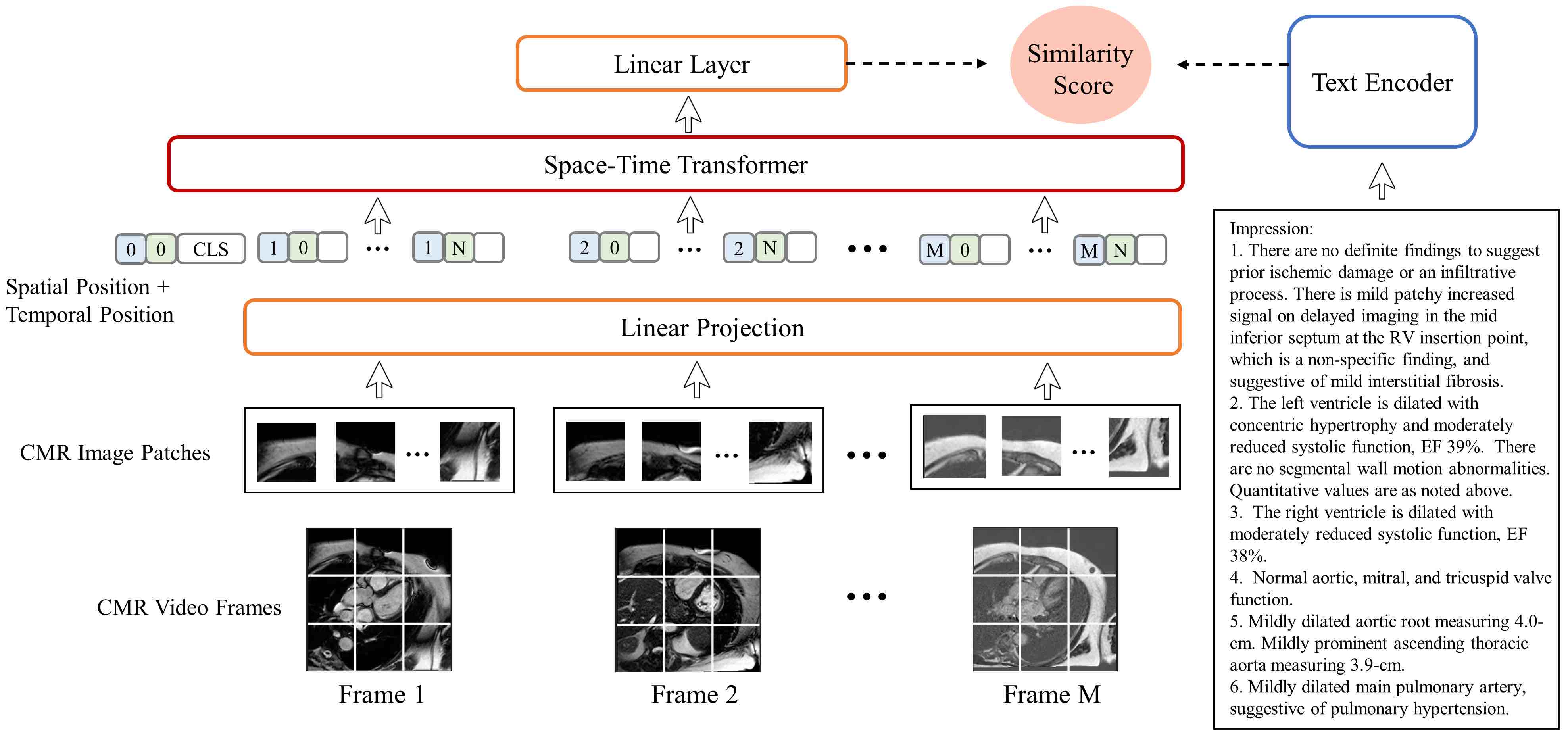
Multimodal Representation Learning of Cardiovascular Magnetic Resonance Imaging
ICML 2023 Workshop on Machine Learning for Multimodal Healthcare Data (ML4MHD 2023)
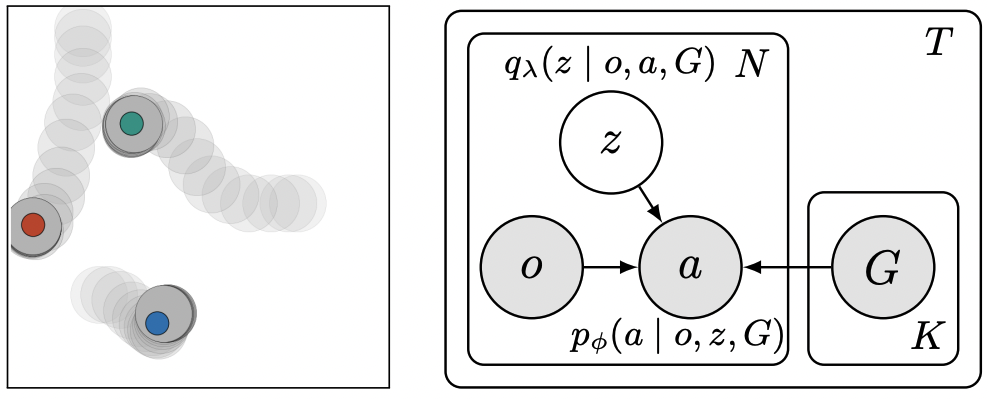
Curriculum Vitae
Click here to download my full CV (last update: Feb 2024).
Education
Carnegie Mellon University
Aug 2020 -- Present
Ph.D.
Specialization: reinforcement learning, deep learning, and robotics
Carnegie Mellon University
Aug 2023 -- Present
M.S.
Specialization: machine learning
Stanford University
Aug 2018 -- June 2020
M.S.
Specialization: robotics, control
Nanyang Technological University, Singapore
Aug 2014 -- June 2018
B.E.
Specialization: aeronautics and space engineering
Internship
Apple, AIML
May 2024 -- Aug 2024
Machine Learning Research Intern
Responsibility: work on robotic foundation models.
Bosch Center for Artificial Intelligence
May 2023 -- Aug 2023
Machine Learning Research Intern
Responsibility: developed a safety-critical scenenario generation algorithm for self-driving vehicles testing.
Flexiv Robotics Ltd.
Aug 2018 -- June 2020
System Engineering Intern
Responsibility: developed a robot evaluation platform in Simulink and a communication protocal in C++ for fast prototyping.
Agency for Science, Technology and Research, Singapore
Jan 2017 -- June 2017
Research Assistant
Responsibility: designed a mobile robot that can change its morphology according to the environment constraints.
Academic Services
Reviewer
- Conference: NeurIPS, ICML, ICLR, AISTATS, ICASSP, CVPR
- Journal: TPAMI, IJCV